Pinpointing Breast Cancer From a Bioengineering Perspective
- Max Dang Vu
- Apr 18, 2022
- 10 min read
Updated: Apr 21, 2022
By Max Dang Vu
In 2020, around 2.3 million women globally were diagnosed with breast cancer, the most people for any cancer type. Simultaneously, almost 685,000 women died from the disease [1]. Breast cancer treatment involves locating tumours early and completely removing them through surgery. To enable this, tumour positions are first analysed and identified across medical images acquired from different diagnostic procedures. Based on these analyses and palpations, the surgeon marks the location to perform a tumour excision. But what are the clinical challenges of breast cancer diagnosis & treatment, and how can we help address them from a bioengineering perspective? This article highlights these challenges and reviews state-of-the-art biomechanical approaches to help find solutions to this question.
Clinical breast cancer diagnosis and treatment procedures
Breast cancer diagnosis involves three imaging procedures: X-ray mammography, magnetic resonance imaging (MRI), and second-look ultrasound (Figure 1). A challenge with this is finding the correspondence of tumour positions between the different procedures because breast tissues undergo large displacements with small changes in patient positioning. The patient stands upright during X-ray mammography, with two plates compressing their breasts to achieve near-uniform distribution of internal tissues [2]. However, tumours and normal breast tissue can appear similar on mammograms, increasing the difficulty in differentiating them [3]. MRI is more effective at discriminating lesions from breast tissue due to the high-resolution contrast between soft tissues [4]. Patients lie face-down (prone position) as gravitational forces separate out tissues in the breast [5]. However, while MRI has high sensitivity, it also has low specificity, making it challenging to differentiate lesion types. This can result in unnecessary biopsies to confirm the presence or absence of tumours. Second-look ultrasound can supplement MRI by visualising lesions in real-time and help catch early-stage cancers. Clinicians apply a handheld high-frequency transducer probe over the patient's breast as they lie face-up (supine position) and tilted to one side to obtain these images [3]. Ultrasound, however, has poor cancer detection sensitivity and is best used to supplement MRI [6].

Figure 1: Breast cancer diagnostic images are taken via X-ray mammography in the standing position (a), followed by MRI in the prone (facedown) position (b) and second-look ultrasound in the supine (face-up) position and tilted to one side (c). Image a is obtained from Monkey Business - stock.adobe.com. Image b is from siemens.com/press. Image c is from Luisandres - stock.adobe.com.
Clinicians typically treat diagnosed tumours by surgically removing them, either through lumpectomy plus radiation or a mastectomy [7]. The intention is to remove tumours altogether to minimise cancer recurrence and optimise survival chances [8]. A lumpectomy eliminates tumours along with small amounts of surrounding healthy tissues to conserve as much of the breast as possible. Follow-up radiation ensures any leftover cancer cells are eliminated or shrink in size for future removal. A mastectomy removes the entire breast when previous treatment strategies are ineffective for patients. This prompts the need for tools that assist in accurate tumour localisation and minimise the removal of healthy breast tissue in treatment.
State-of-the-art development in the literature
These clinical challenges have motivated the development of physics-driven computational models that predict breast biomechanics. The models can simulate motion under gravity loading from the prone position to the supine position, where surgical treatment is performed. This enables radiologists and surgeons to track the features of interest during clinical procedures [9], [10]. Breast biomechanical models are built using the Finite Element Method (FEM), which divides a geometry of interest into a mesh composed of many smaller elements. Partial differential equations describing the breast's mechanical behaviour are solved to predict the breast tissue deformation [11]. Deformation describes an object's changing shape and size in space under applied forces.
Obtaining accurate predictions from biomechanical models requires identifying the mechanical properties of breast tissue. These properties provide insights into breast tissue composition as its underlying architecture and biological environment dictate their mechanical moduli or stiffness [12]. The breast is internally composed of adipose and fibroglandular tissues [13], and their reported stiffnesses vary with different mechanical loading conditions and experimental protocols used for their identification [14]. From the testing of excised samples of tissues (ex-vivo), the general observation is that fibroglandular tissues are 1 to 6.7 times stiffer than adipose tissue [13], and tumours have significantly higher stiffnesses that increase with cancer growth [15]. Researchers typically assess tissue stiffness in-vivo to avoid tissue removal and subsequent damage that may alter their mechanical behaviour during testing [16]. However, identifying mechanical properties in-vivo requires a rich dataset acquired either from MR imaging of the breasts in multiple positions or capturing surface deformation of the breast under indentation using multi-camera systems [10], which is highly challenging to obtain. Therefore, the validation of these identification methods is typically conducted first by performing experiments on soft silicone gel phantoms. These can be moulded into different shapes, such as rectangular beams or the breast [17-18].
At this point, tumours have been identified via imaging, and their locations predicted using biomechanics during surgery. This information is to be communicated to clinicians to assist in tumour localisation. Existing approaches display these predictions on a 2D interface [10]. However, such communication should be more intuitive to improve treatment outcomes. Head-mounted holographic augmented reality (AR) systems have the potential to visualise tumour locations directly on patients during clinical procedures [19-20]. These systems have been successfully trialled in orthopaedics [21] and neurosurgery [22] because the tissues of interest deform minimally during interventions. Perkins (2017) [19] found that aligning holograms to the breast is far more challenging, as the breast significantly deforms with small positional changes. However, proof-of-concept studies combining AR systems with biomechanical models by Gouveia (2021) [20] demonstrated some promise. Clinicians could visualise the identified tumours from diagnostic images on their view of patients before interventions.
Clinical translation challenges of these developments
While proof-of-concept demonstrations have been developed, researchers must address the following challenges to enable routine use of this technology in the clinic. Firstly, state-of-the-art FEM simulations can take 30 seconds or longer to run [23], which is slower than the 60 frames-per-second required to reduce nausea and disorientation [24]. A proposed solution is surrogate models, which uses machine learning to accelerate the evaluation of the models but maintain similar accuracy to FEM models. Studies using decision trees, randomised trees, and random forest models have enabled breast tissue deformation predictions under compression in about 0.15 seconds [25]. However, these approaches require training surrogate models offline for each patient that will be considered, which can be time-consuming. Studies in the breast biomechanics literature have utilised trained models from a previous problem to predict the mechanical behaviour of a new dataset [26]. Secondly, estimating mechanical properties is also a computationally intensive procedure, often taking hours to complete. The model parameters that describe, for example, the stiffness of breast tissues are tuned iteratively to best match measured breast shape under known loading conditions [14]. Clinical use requires this process to be much faster. Thirdly, clinicians need their AR headset to align the 3D hologram dynamically with the object of interest as they move their heads around. Studies in the literature have assumed the breast is rigid, making it difficult to align the model hologram with the real breast [19-20]. The breast must align with a deformable model that incorporates mechanical properties to account for how even small changes in patient positioning can alter breast shape.
Objectives of the PhD
The challenges above have motivated me to develop an integrated physics-driven AR software platform that will provide navigational guidance to clinicians for tumour localisation. My platform will extend an automated clinical image analysis workflow developed by the Breast Biomechanics Research Group at the Auckland Bioengineering Institute [10] to align diagnostic images directly onto a clinician's view of a patient during breast cancer treatment procedure (Figure 2).
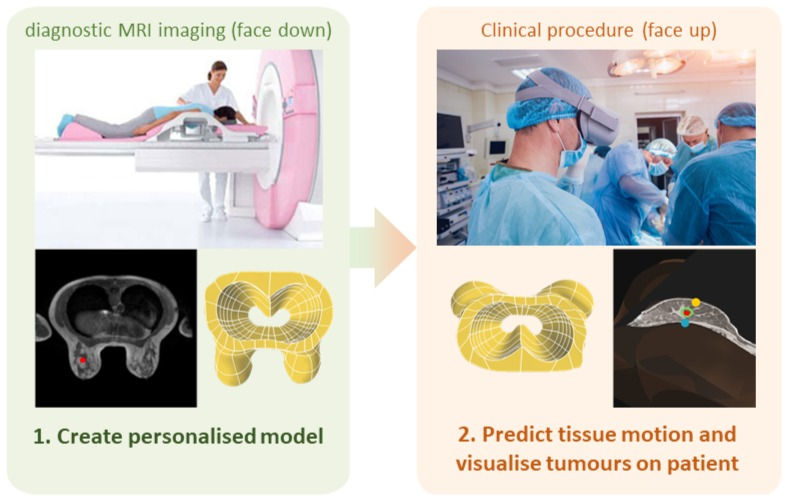
Figure 2: My proposed physics-driven AR platform will leverage an automated clinical workflow for breast cancer image analysis [10]. The workflow builds personalised biomechanical models of the breast from diagnostic MRI and visualises breast tissue displacements in near real-time during clinical procedures performed in supine. In addition to technical developments for accelerating the workflow and identifying mechanical properties of the breast, my work will replace the GUI with an AR workflow that aligns diagnostic images to the clinician's view of patients. Images are obtained from Romaset - stock.adobe.com
One of my platform's key features is near real-time simulation of breast tissue motion using surrogate models that incorporate information from population-based breast shape analyses. This will enable the surrogate models to provide predictions without time-consuming offline training. I will also integrate surrogate models developed in-house [27] with skin surface measurements from sensors on AR headsets (Figure 3) to enable estimation of breast tissue stiffness under known loading conditions (changes in an individual’s posture which changes the gravity loading conditions the breast experiences). The platform will integrate these developments with fiducial markers placed on the breast surface, and shape measurements from AR headset sensors to enable dynamic alignment of biomechanics simulations to the patient. The platform will apply the tissue displacements predicted by the mechanics simulations to the diagnostic prone MRI to help clinicians visualise how the internal tissues change shape in the supine position. This will help clinicians co-locate regions of interest across modalities e.g. between MRI and second-look ultrasound images. I will develop the platform in my first year and incorporate it into a state-of-the-art AR headset (Microsoft HoloLens 2 shown in Figure 3). The platform's accuracy for predicting supine tumour locations will be validated during platform development on soft silicone gel phantoms with tumour-like inclusions. In subsequent years, I will evaluate the platform's performance on patients in a series of clinical pilot studies.

Figure 3: A diagram of the Microsoft Hololens 2 AR headset to be embedded with the developed software platform. Clinicians will be wearing these during procedures to visualise the predicted tumour locations onto patients directly. This image is from the Microsoft News Center Image Gallery.
Breast cancer research is fast becoming an interdisciplinary field. Whether it is medical image registration, large deformation mechanics modelling, or computer vision, research opportunities are growing to address the field's significant challenges. I hope my work contributes to increasing the accuracy and efficiency of breast cancer treatment, improving health outcomes, and saving more lives.
Acknowledgements
External editors
I want to thank Dr Prasad Babarenda Gamage, Dr Gonzalo Maso Talou, and Dr Huidong Bai for their guidance throughout the editing process, for approving the article proposal, and for supervising my PhD study in the ABI's Breast Biomechanics Research Group.
Funding
The ABI's Breast Biomechanics Research Group is grateful for the funding we have received from the University of Auckland Foundation, the New Zealand Breast Cancer Foundation, and the New Zealand Ministry of Business, Innovation and Employment that has supported our research. I would also like to thank The University of Auckland for awarding me a Doctoral Scholarship to support my PhD study financially.
References
H. Sung et al., “Global Cancer Statistics 2020: GLOBOCAN Estimates of Incidence and Mortality Worldwide for 36 Cancers in 185 Countries,” CA: A Cancer Journal for Clinicians, vol. 71, no. 3, pp. 209–249, 2021, doi: 10.3322/caac.21660.
A. Mîra, A. K. Carton, S. Muller, and Y. Payan, “A biomechanical breast model evaluated with respect to MRI data collected in three different positions,” Clinical Biomechanics, vol. 60, no. February, pp. 191–199, 2018, doi: 10.1016/j.clinbiomech.2018.10.020.
R. F. Brem, M. J. Lenihan, J. Lieberman, and J. Torrente, “Screening breast ultrasound: Past, present, and future,” American Journal of Roentgenology, vol. 204, no. 2, pp. 234–240, 2015, doi: 10.2214/AJR.13.12072.
L. Lebron-Zapata and M. S. Jochelson, “Overview of Breast Cancer Screening and Diagnosis,” PET Clinics, vol. 13, no. 3, pp. 301–323, Jul. 2018, doi: 10.1016/j.cpet.2018.02.001.
R. M. Mann, N. Cho, and L. Moy, “Breast MRI: State of the art,” Radiology, vol. 292, no. 3, pp. 520–536, 2019, doi: 10.1148/radiol.2019182947.
V. Y. Park, M. J. Kim, E. K. Kim, and H. J. Moon, “Second-look US: How to find breast lesions with a suspicious MR imaging appearance,” Radiographics, vol. 33, no. 5, pp. 1361–1375, 2013, doi: 10.1148/rg.335125109.
A. G. Waks and E. P. Winer, “Breast Cancer Treatment: A Review,” JAMA, vol. 321, no. 3, p. 288, Jan. 2019, doi: 10.1001/jama.2018.19323.
M. S. Abrahimi, M. Elwood, R. Lawrenson, I. Campbell, and S. Tin Tin, “Associated Factors and Survival Outcomes for Breast Conserving Surgery versus Mastectomy among New Zealand Women with Early-Stage Breast Cancer,” IJERPH, vol. 18, no. 5, p. 2738, Mar. 2021, doi: 10.3390/ijerph18052738.
A. W. C. Lee, V. Rajagopal, T. P. Babarenda Gamage, A. J. Doyle, P. M. F. Nielsen, and M. P. Nash, “Breast lesion co-localisation between X-ray and MR images using finite element modelling,” Medical Image Analysis, vol. 17, no. 8, pp. 1256–1264, Dec. 2013, doi: 10.1016/j.media.2013.05.011.
T. P. B. Gamage et al., “An automated computational biomechanics workflow for improving breast cancer diagnosis and treatment,” Interface Focus, vol. 9, no. 4, pp. 1–12, 2019, doi: 10.1098/rsfs.2019.0034.
O. C. Zienkiewicz, R. L. Taylor, and D. Fox, The Finite Element Method for Solid and Structural Mechanics, Seventh. Oxford: Butterworth-Heinemann, 2013. doi: 10.1016/C2009-0-26332-X.
M. P. Nash and P. J. Hunter, “Computational mechanics of the heart. From tissue structure to ventricular function,” Journal of Elasticity, vol. 61, no. 1–3, pp. 113–141, 2000, doi: 10.1023/A:1011084330767.
D. E. McGhee and J. R. Steele, “Breast biomechanics: What do we really know?,” Physiology, vol. 35, no. 2, pp. 144–156, 2020, doi: 10.1152/physiol.00024.2019.
T. P. Babarenda Gamage, P. M. F. Nielsen, and M. P. Nash, “Clinical Applications of Breast Biomechanics,” in Biomechanics of Living Organs: Hyperelastic Constitutive Laws for Finite Element Modeling, Elsevier Inc., 2017, pp. 215–242. doi: 10.1016/B978-0-12-804009-6.00010-9.
N. G. Ramião, P. S. Martins, R. Rynkevic, A. A. Fernandes, M. Barroso, and D. C. Santos, “Biomechanical properties of breast tissue, a state-of-the-art review,” Biomechanics and Modeling in Mechanobiology, vol. 15, no. 5, pp. 1307–1323, 2016, doi: 10.1007/s10237-016-0763-8.
P. Elsner, E. Berardesca, and K.-P. Wilhelm, Eds., Bioengineering of the Skin: Skin Biomechanics, Volume V, 0 ed. CRC Press, 2001. doi: 10.1201/b14261.
V. Rajagopal, J. H. Chung, D. Bullivant, P. M. F. Nielsen, and M. P. Nash, “Determining the finite elasticity reference state from a loaded configuration,” International Journal for Numerical Methods in Engineering, vol. 72, no. 12, pp. 1434–1451, 2007, doi: 10.1002/nme.2045.
T. P. Babarenda Gamage, V. Rajagopal, M. Ehrgott, M. P. Nash, and P. M. F. Nielsen, “Identification of mechanical properties of heterogeneous soft bodies using gravity loading,” International Journal for Numerical Methods in Biomedical Engineering, vol. 27, no. 4, pp. 391–407, 2011, doi: 10.1002/cnm.1429.
S. L. Perkins, M. A. Lin, S. Srinivasan, A. J. Wheeler, B. A. Hargreaves, and B. L. Daniel, “A Mixed-Reality System for Breast Surgical Planning,” Adjunct Proceedings of the 2017 IEEE International Symposium on Mixed and Augmented Reality, ISMAR-Adjunct 2017, pp. 269–274, 2017, doi: 10.1109/ISMAR-Adjunct.2017.92.
P. F. Gouveia et al., “Breast cancer surgery with augmented reality,” Breast, vol. 56, pp. 14–17, 2021, doi: 10.1016/j.breast.2021.01.004.
N. Navab, S.-M. Heining, and J. Traub, “Camera Augmented Mobile C-Arm (CAMC): Calibration, Accuracy Study, and Clinical Applications,” IEEE Trans. Med. Imaging, vol. 29, no. 7, pp. 1412–1423, Jul. 2010, doi: 10.1109/TMI.2009.2021947.
R. M. Comeau, A. F. Sadikot, A. Fenster, and T. M. Peters, “Intraoperative ultrasound for guidance and tissue shift correction in image-guided neurosurgery,” Med. Phys., vol. 27, no. 4, pp. 787–800, Apr. 2000, doi: 10.1118/1.598942.
L. Han et al., “A nonlinear biomechanical model based registration method for aligning prone and supine MR breast images,” IEEE Transactions on Medical Imaging, vol. 33, no. 3, pp. 682–694, 2014, doi: 10.1109/TMI.2013.2294539.
A. Vovk, F. Wild, W. Guest, and T. Kuula, “Simulator sickness in Augmented Reality training using the Microsoft HoloLens,” Conference on Human Factors in Computing Systems - Proceedings, vol. 2018-April, pp. 1–9, 2018, doi: 10.1145/3173574.3173783.
F. Martínez-Martínez et al., “A finite element-based machine learning approach for modeling the mechanical behavior of the breast tissues under compression in real-time,” Computers in Biology and Medicine, vol. 90, no. September, pp. 116–124, 2017, doi: 10.1016/j.compbiomed.2017.09.019.
A. Mendizabal, E. Tagliabue, J.-N. Brunet, D. Dall’Alba, P. Fiorini, and S. Cotin, “Physics-Based Deep Neural Network for Real-Time Lesion Tracking in Ultrasound-Guided Breast Biopsy,” Computational Biomechanics for Medicine, pp. 33–45, 2020, doi: 10.1007/978-3-030-42428-2_4.
G. D. Maso Talou, T. P. Babarenda Gamage, M. Sagar, and M. P. Nash, “Deep Learning Over Reduced Intrinsic Domains for Efficient Mechanics of the Left Ventricle,” Frontiers in Physics, vol. 8, pp. 1–14, 2020, doi: 10.3389/fphy.2020.00030.
Comments